Navigating the Ethical Minefield of Big Data: Balancing Innovation and Privacy
- Aanchal
- May 6, 2024
- 3 min read
Updated: May 10, 2024
The dizzying growth of the global datasphere is something we've witnessed firsthand. In the digital era, data analytics has become a cornerstone of strategic decision-making across industries. The potential for data-driven breakthroughs is immense, but as researchers, we've got to acknowledge the flip side – the increasing ethical complexities that come with big data analytics.
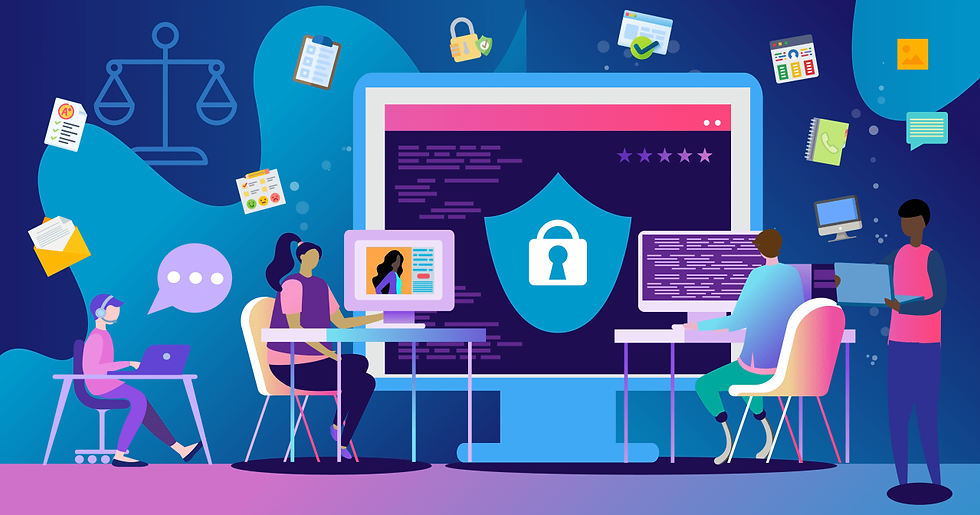
Statistical Insights and Technological Advancements
The data landscape is characterized by rapid growth and technological advancements:
Data Growth: The total volume of data created globally is expected to reach 149 zettabytes by 2024, illustrating the expanding scope of data management challenges.
AI and ML Adoption: Over 70% of financial institutions have integrated AI to enhance risk management and fraud detection, showcasing the growing reliance on advanced analytics.
Impact of Data Quality: Poor data quality costs organizations an average of $12.8 million annually, highlighting the importance of robust data management practices.
Data Breaches and Privacy Concerns: With a 30% increase in data breaches over the past year, the need for stringent security measures has never been more urgent.
ROI from Analytics: Organizations investing in analytics report an average 8-10% increase in revenue and a 10% reduction in costs, underscoring the tangible benefits of data-driven strategies.
Transparency: Beyond a Buzzword
It's easy to throw the word 'transparency' around, but we need actionable steps. This isn't just about compliance – it's about establishing trust with the people whose data ultimately fuels our models. How we communicate data collection and usage matters. Privacy by design has to be our default, from the earliest stages of any project. Yes, techniques like anonymization have a role, but let's be honest, they aren't foolproof. That's why we need to keep a close eye on differential privacy – it offers a genuinely innovative privacy-utility tradeoff.
Confronting the Challenge of Bias
We've all seen the headlines about biased algorithms, but it hits differently when you're the one staring at the results. Data can be a mirror to societal inequalities, and if we're not careful, our models become amplifiers. This isn't a theoretical concern, mind you. Facial recognition, hiring algorithms, even healthcare risk models – the potential for harm is real. That's why techniques for fairness-aware machine learning aren't optional anymore; they're a necessity. And this is where I think explainable AI (XAI) is a real game-changer. Tools like LIME and SHAP don't just help justify a decision to a regulator, they force us to take a hard, honest look at the mechanics of our own models.
Security and Governance: The Non-Negotiables
Every data breach is a stark reminder that security isn't a 'nice to have,' it's the bedrock of ethical data science. Of course, strong encryption and access controls are hygiene factors. What worries me more is a lack of solid data governance within organizations. We need clear, consistently enforced frameworks, not just knee-jerk reactions to the latest privacy legislation. And regular audits aren't about ticking boxes, they're about developing the reflexes that help us spot potential ethical breaches early.
Innovation at the Privacy Frontier
The ethics of data science isn't about restricting innovation, it's about finding smarter ways to do things. Federated learning, for one, offers a way around some of the thorniest privacy problems. By training models across data silos without sharing raw data, it opens up avenues for collaboration that were previously off-limits. Differential privacy, too, is one to watch - the ability to analyze datasets while mathematically guaranteeing individual privacy could be a watershed moment.
People, Not Just Pixels
Here's the thing, ethical data science isn't purely technical. We need to foster a culture within our teams and organizations where the potential impacts of our work are always top of mind. Educating and empowering individuals about their data rights is also part of the equation. This is a field where researchers, businesses, and policymakers all need a seat at the table.
Let's face it, big data analytics is a powerful force, and with great power… well, you know the rest. The ethical challenges we're grappling with won't have easy answers. But, by pushing for transparency, confronting bias head-on, prioritizing privacy, and staying attuned to innovation, we'll steer this technology towards a future that truly benefits us all.